This is going to be a long post, so I’m going to front-load the top line results (with a little bit of history) and then get into a longer discussion of some of the details after that for anyone who is interested.
One of the earliest and arguably still most important discoveries in hockey statistics is that, at the team level, past goal scoring is not a very good predictor of future scoring. Early writers in hockey statistics discovered that you could do a better job of predicting how well teams would score at even strength by looking at shot attempts instead of goals. Over time many people began to argue that because there are differences in the quality of shots, improvements could be made by adjusting each shot for its likelihood of becoming a goal, based on factors like how close to the net the shot is and what type of shot was taken. We call this adjusted measure Expected Goals, or xG.
The first example of an xG model that I’m aware of was created by former Florida Panthers analyst Brian MacDonald back in 2012. Unfortunately, his research does not appear to be available any longer. [UPDATE: Since publishing this article, it’s come to my attention that statistics like expected goals go back to at least 2006, prior to the NHL first publishing shot location data!] [UPDATE 2: And an even older xG model from Alan Ryder in 2004.] It wasn’t until 2015 that an xG model gained wider public attention, when Dawson Sprigings (who now works for the Colorado Avalanche) and Asmae Toumi collaborated on a model for Hockey Graphs (for lack of a better name, I will refer to this as the DA model for the rest of this post). According to their article, expected goals are better at predicting future results than Corsi is. This was the breakthrough that many people had been waiting for, a metric that tried to account for the quality of shots rather than just their quantity.
In the years since then, a number of people have created their own xG models. While the raw data for the DA model is not publically available, the makers of more recent metrics have put the data online so that anyone can use it. While it is impossible to evaluate every model that’s out there, I collected data from three of the most commonly-used public models to do some new testing of these metrics. The data I’m using comes from Moneypuck, Evolving Hockey, and Natural Stat Trick.
Results
Using those three models, I collected 5v5 data from every 82-game regular season game played since 2007, which is the first season for which we have modern statistics. (Moneypuck only has data from 2008 on, but this does not materially affect the results, as you’ll see.) I’ve exclusively used score-adjusted metrics for reasons that are well-established.
To see how predictive each web site’s model is of future results, I split each season into two halves, the first 41 games for each team, and the second 41 games for each team. I tested how well you could predict a team’s goal ratio (GF%) in the second half of the season based on their results in the first half of the season in four different metrics: Corsi (all shot attempts), xG (shots on net and missed shots, adjusted for shot quality), scoring chances (a hybrid approach that counts all shots from a “scoring chance” area of the ice, excluding shots from further away), and goals. Only Natural Stat Trick tracks scoring chances, but since I’ve done a bit of work with scoring chances in the past, and since I had the data anyway, I’ve included it here. I’m using a measurement called “r-squared“, which tells you how well one variable predicts a second variable (more or less). An r-squared of 1.0 would mean that the first variable explains the variance in the second variable 100%. A value closer to zero means that the first variable does not have a strong relationship to the second.
So how well does each metric do at predicting future goal scoring, including every 82-game season back to 2007?
Site | GF>GF | SCF>GF | xGF>GF | CF>GF |
NST | 0.09 | 0.18 | 0.15 | 0.19 |
EH | 0.09 | – | 0.14 | 0.19 |
MP | 0.10 | – | 0.14 | 0.18 |
Contrary to what was reported for the DA model back in 2015, I find that no matter what model you use, Corsi is always better at predicting future results than expected goals are. xG under-performs Corsi by a very similar amount in all three models that I tested. Given three different models created for three different sites have produced nearly identical results, I think I’m reasonably confident in saying that Corsi is simply a better measurement of team quality, and hockey fans should probably stop using expected goals, at least at the team level (it’s possible they’re of more value at the player level, but I haven’t tested that). The results here are too consistent for me to believe that it’s just a case of one model having poor design, or something of that nature.
Why are these results so different than the DA model? I’m not really sure, but since that model isn’t public and it’s not possible for me to test it, I can only say that I’m not able to reproduce their results with the models that I do have access to.
There has been some discussion in the past that the NHL’s shot location data is incomplete prior to the 2009-10 season, and that metrics adjusted based on shot location may be more reliable than you would conclude if you look at all available seasons. For reasons I will get to in a moment that seems plausible based on some other things I’ve found, so let’s try this again throwing out the first two seasons (2007 and 2008).
Site | GF>GF | SCF>GF | xGF>GF | CF>GF |
NST | 0.10 | 0.21 | 0.17 | 0.21 |
EH | 0.10 | – | 0.16 | 0.21 |
MP | 0.10 | – | 0.15 | 0.20 |
As you can see, this improves the predictivity of all four statistics, but, crucially, it does not change the relative value of the statistics. Corsi still comes out well ahead of all three xG models.
Digging Deeper
While going through the numbers in a bit more detail, I noticed something interesting: Corsi’s advantage over the other statistics has been shrinking over time. Let’s take a look at these statistics again, but break them down a bit differently. Let’s do a rolling average in 5 year blocks. From here on out I will only be using data from Natural Stat Trick, partly because it has the xG model with the best results, partly because I also want to take a look at scoring chances. For what it’s worth, I’ve looked at these questions with the EH data and get the same results, so I’m sticking to one model here just to keep things simple.
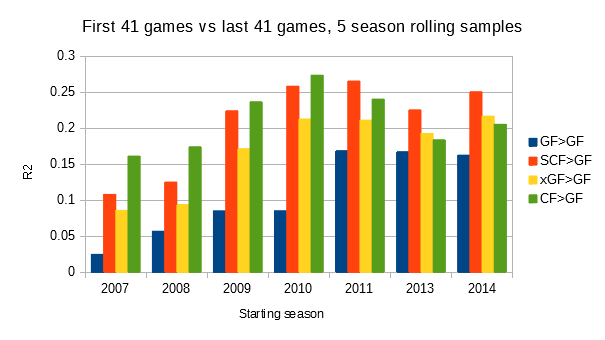
You can see the big jump in predictivity for the location-specific stats (SCF and xGF) starting in 2009, which lines up with the previous discussion that there may be problems with the location data prior to that point.
Also of note is that scoring chances have been better at predicting future goals than Corsi has over nearly a decade now. This lines up with research I published two years ago, and it’s interesting to see that trend continue. Expected goals have also caught up to Corsi and even very slightly passed it in predictivity, although the gap is very small, and at any rate scoring chances are more predictive than expected goals at every point measured.
For the sake of argument let’s say that the data since 2009 is of better quality than the prior data. I don’t think I’m qualified to judge that and so I will leave it to others who know the data better than I do, but let’s go with it for the sake of argument. Since 2009, scoring chances and Corsi have been comparably predictive, and for nearly a decade scoring chances have in fact provided better predictive value. I think there is a pretty good argument at this point that scoring chances are actually the superior metric. However, I would also argue that at this point there is no argument for using expected goals for evaluating teams. Scoring chances are consistently better by a reasonably large margin during the entire period for which we have data. There is no point at which xG is the most predictive of the statistics I’ve looked at.
Splitting Out Offence and Defence
I’m going to get into a point I’ve made in my previous research on scoring chances, and something I’ve been arguing pretty consistently since that time. It’s something that I’ve not been able to convince a lot of other people of, but I’m going to take another crack at it here:
Offence and defence are not two sides of the same coin, and we probably shouldn’t treat them the same way.
It is true that scoring chances have been better at predicting team level goal ratio over the past decade or so than Corsi has. But it is important to note that this improvement is based entirely on being better at predicting goal scoring. Corsi is still better, by quite a large margin, at predicting goal prevention.
Let’s look specifically at the most recent 5 seasons, the time period when the advantage for scoring chances has been at its largest.
CF60>GF60 | SCF60>GF60 |
0.24 | 0.29 |
CA60>GA60 | SCA60>GA60 |
0.20 | 0.14 |
It’s worth noting that this matches what I’ve found in my previous research on scoring chances (it is also true of expected goals).
You will have a better idea which teams are good at scoring goals by looking at quality-adjusted metrics, but you’ll get a better idea about which teams are good at preventing goals by looking at pure shot attempts. We don’t have any good metrics at the moment that account for this distinction, but I think it’s a pretty promising avenue for future research (and something I’m hoping to find time this off-season to tackle myself).
Conclusion
To sum up in a few points:
- Expected goals are not the most predictive measure over any time period for which we have shot location data.
- Over the full time period available, Corsi is the most predictive metric.
- In more recent years scoring chances have been the best metric, although it’s not clear whether that’s due to something fundamental changing in the results or if it’s just natural variation over time.
- You’re better off describing offence and defence using different metrics, because shot quality seems to matter more on offence than on defence.
Leave a comment